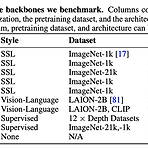
NeurIPS 2023, Arxiv Link What they didThey propose "Battle-of-the-Backones"(BoB), benchmarking a diverse suite of (vision) pre-trained models, including classic ImageNet-trained CNN, vision-language models (perhaps CLIP). The target tasks are OOD-generalization, classification, object-detection and Image retrieval.Key observationswith more than 1,500 training runs, they found that CNN pretrained..
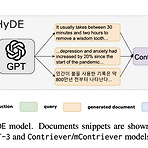
ACL 2023. 이긴 하지만 아카이브에는 2022에 올라왔음.Super-simple backgroundRAG (Retrieval Augmented Generation) is commonly used to complement the hallucination of LLMs.to find the proper documents (here, we call target document) for given queries, the "contriever" is used.the contriever can be an text-encoder model such as T5 or BERT.the target document can be searced with the encoded feature by the contriever ..
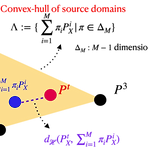
Here I introduce the generalization error bound of the Domain generalization problem, which is the test domain—or style, sometimes—differs from the training domain.PreliminariesNotations$X \in \mathcal{X} \subset \mathbb{R}^d, Y\in \mathcal{Y} \subset \mathbb{R}$ : Common input and target space$P^i_{XY}$: Data distribution of the i'th domain$S^i\sim P^i_{XY}$: Samples for the i'th domain$\epsilo..
- Total
- Today
- Yesterday
- Transformer
- loss-weighting
- multiheadattention
- DeepLearning
- Theme
- generativemodeling
- ICML
- diffusion
- vscode
- MachineLearning
- domaingeneralization
- LLM
- flowmatching
- 몽중식
- 프렌밀리
- deeplearning4science
- icml2024
일 | 월 | 화 | 수 | 목 | 금 | 토 |
---|---|---|---|---|---|---|
1 | 2 | 3 | 4 | 5 | 6 | 7 |
8 | 9 | 10 | 11 | 12 | 13 | 14 |
15 | 16 | 17 | 18 | 19 | 20 | 21 |
22 | 23 | 24 | 25 | 26 | 27 | 28 |
29 | 30 |