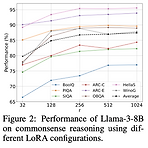
ICLR2025논문의 내용과 상관없이, 제가 이야기해보고 싶은 부분은 이탤릭체로 남깁니다.texts with Italic represent parts that are not the part of the original paper, but rather my personal thoughts or additionsLimitation of the LoRALoRA and most of its variants do not perform well when applied to complex tasks—such as commonsense reasoning.This degradation might be inevitable since the additional trainable parameters are “lower-ran..
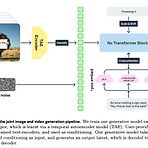
IntroModern foundation models for image and video generation are often closed-source, with many technical details remaining unavailable to the public—this is especially true for video generation models. (In my view, this closed-source trend is even more pronounced than in the LLM domain.). For example, we know very little, if anything, about the technical specifics behind models like Runway Gen3..
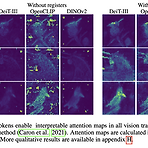
이 포스트는 Academic-reels와 특집 어딘가에 있는... shorts는 아니지만 그렇다고 10분짜리 비디오도 아닌 그런 구성입니다. 정리본 같은 거랄까요되게 Scientific 하게 잘 쓰인 논문입니다. 점수가 매우 높아요. 흥미로운 가설을 설정했고, 가설을 support 하는 좋은 관측들을 했고, 그에 따른 simple-but-effective 한 방법을 제시합니다. 글도 매우 잘 써진 것 같고요.별 5.FAIR, Meta, ICLR 2024 OralObjective and motivation(Our objective) Vision transformer의 마지막 attention layer를 visualize 해보면, 위와 같은 “abnormal patch“ 가 보인다 (semantically..
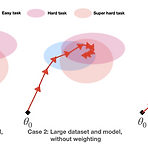
들어가며딥러닝 연구에서, 다양한 문제들이 기존 loss-function에 sample별로 특정 weight를 부과하는 방법 (Loss-reweighting)으로 풀어져 왔었다. 직관적으로 생각했을 때, 이러한 방식은 "어떤 샘플들을 중점적으로 학습시켜야 하는가?"라는 식의 물음이 이어지는 문제를 풀어내는 도구가 될 수 있었다. 따라서 저런 물음이 많이 나오게 되는 Domain-generalization 문제---어떤 데이터 도메인 (e.g. 그림, 사진, 선화, 애니메이션)을 중점적으로 학습시킬까?---, multi-task 문제---여러 task를 동시에 푸는 모델이 어떤 task (depth estimation, object-detction, segmentation)를 중점적으로 학습해야 할 까?,I..
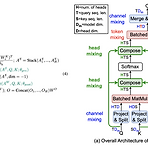
ICML2024; OralMotivation: the limitation of the MHA (Multi-Head-Attention)MHA는 Dimension 이 head의 수로 나누어지는 특성상 low-rank bottleneck이 생길 수 있다.MHA에서는 head-redundancy가 생길 수 있다. (여러 head들끼리 비슷한 동작을 할 수 있다)결론적으로, 이 문제들을 해소할 vanilla MHA의 훌륭한 대체제를 찾고 싶다.본 논문에서는, 위와 같은 multi-head-attention의 문제를 "attention-score composition"을 통해 풀고자 한다. 논문에 걸쳐 어떻게 attention-score composition이 위 문제에 도움이 될 수 있는지를 설명하고, 어떤 방식..
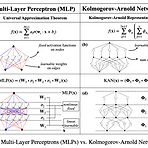
Arxiv Link Contributions: propose a novel architecture which possibly be better MLP-alternative, having surely better interpretability and probably better accuracy. the new architecture has opened up the possibility of learning activation function itself instead of the traditional approach of learning weights.Background: Kolmogorov Arnold Representation theoremMachine learning에서, MLP는 일반적으로 univ..
- Total
- Today
- Yesterday
- 이문설농탕
- diffusion
- ICML
- domaingeneralization
- multiheadattention
- Transformer
- flowmatching
- deeplearning4science
- vscode
- 프렌밀리
- MachineLearning
- finetuning
- DeepLearning
- loss-weighting
- generativemodeling
- 몽중식
- LLM
- icml2024
- Theme
일 | 월 | 화 | 수 | 목 | 금 | 토 |
---|---|---|---|---|---|---|
1 | 2 | 3 | 4 | 5 | 6 | 7 |
8 | 9 | 10 | 11 | 12 | 13 | 14 |
15 | 16 | 17 | 18 | 19 | 20 | 21 |
22 | 23 | 24 | 25 | 26 | 27 | 28 |
29 | 30 |