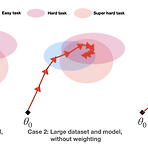
들어가며딥러닝 연구에서, 다양한 문제들이 기존 loss-function에 sample별로 특정 weight를 부과하는 방법 (Loss-reweighting)으로 풀어져 왔었다. 직관적으로 생각했을 때, 이러한 방식은 "어떤 샘플들을 중점적으로 학습시켜야 하는가?"라는 식의 물음이 이어지는 문제를 풀어내는 도구가 될 수 있었다. 따라서 저런 물음이 많이 나오게 되는 Domain-generalization 문제---어떤 데이터 도메인 (e.g. 그림, 사진, 선화, 애니메이션)을 중점적으로 학습시킬까?---, multi-task 문제---여러 task를 동시에 푸는 모델이 어떤 task (depth estimation, object-detction, segmentation)를 중점적으로 학습해야 할 까?,I..
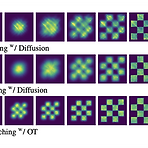
ICLR2023 Notable top 25% (10/8/8/5), Stable diffusion 3Motivation어떤 data-distribution 에서 simple-distribution (e.g. standard gaussian) 으로 변화하는 path (e.g. forward-diffusion process) 를 좀 더 잘 정의해서, 그것의 inverse (image generation via the diffusion model) 또한 더 잘 되도록 하고싶다 Simple diffusion process (adding a “simple“ gaussian noise) leads to rather confined space of sampling probability paths. (라고 표현하고 있는..
- Total
- Today
- Yesterday
- domaingeneralization
- Theme
- ICML
- vscode
- generativemodeling
- 몽중식
- DeepLearning
- 프렌밀리
- diffusion
- LLM
- icml2024
- multiheadattention
- Transformer
- MachineLearning
- flowmatching
- deeplearning4science
- loss-weighting
일 | 월 | 화 | 수 | 목 | 금 | 토 |
---|---|---|---|---|---|---|
1 | 2 | 3 | 4 | 5 | 6 | 7 |
8 | 9 | 10 | 11 | 12 | 13 | 14 |
15 | 16 | 17 | 18 | 19 | 20 | 21 |
22 | 23 | 24 | 25 | 26 | 27 | 28 |
29 | 30 |