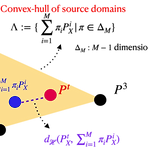
Here I introduce the generalization error bound of the Domain generalization problem, which is the test domain—or style, sometimes—differs from the training domain.PreliminariesNotationsX∈X⊂Rd,Y∈Y⊂R : Common input and target spacePiXY: Data distribution of the i'th domainSi∼PiXY: Samples for the i'th domain$\epsilo..
Showing off studying ML/ML - academic reels
2024. 4. 30. 00:46
공지사항
최근에 올라온 글
최근에 달린 댓글
- Total
- Today
- Yesterday
링크
TAG
- icml2024
- domaingeneralization
- deeplearning4science
- DeepLearning
- LLM
- 이문설농탕
- multiheadattention
- Transformer
- generativemodeling
- finetuning
- diffusion
- MachineLearning
- 몽중식
- vscode
- loss-weighting
- ICML
- 프렌밀리
- flowmatching
- Theme
일 | 월 | 화 | 수 | 목 | 금 | 토 |
---|---|---|---|---|---|---|
1 | 2 | 3 | 4 | 5 | ||
6 | 7 | 8 | 9 | 10 | 11 | 12 |
13 | 14 | 15 | 16 | 17 | 18 | 19 |
20 | 21 | 22 | 23 | 24 | 25 | 26 |
27 | 28 | 29 | 30 |
글 보관함