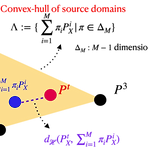
Here I introduce the generalization error bound of the Domain generalization problem, which is the test domain—or style, sometimes—differs from the training domain.PreliminariesNotations$X \in \mathcal{X} \subset \mathbb{R}^d, Y\in \mathcal{Y} \subset \mathbb{R}$ : Common input and target space$P^i_{XY}$: Data distribution of the i'th domain$S^i\sim P^i_{XY}$: Samples for the i'th domain$\epsilo..
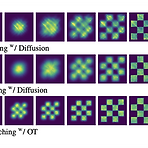
ICLR2023 Notable top 25% (10/8/8/5), Stable diffusion 3Motivation어떤 data-distribution 에서 simple-distribution (e.g. standard gaussian) 으로 변화하는 path (e.g. forward-diffusion process) 를 좀 더 잘 정의해서, 그것의 inverse (image generation via the diffusion model) 또한 더 잘 되도록 하고싶다 Simple diffusion process (adding a “simple“ gaussian noise) leads to rather confined space of sampling probability paths. (라고 표현하고 있는..
- Total
- Today
- Yesterday
- Transformer
- DeepLearning
- MachineLearning
- ICML
- domaingeneralization
- 프렌밀리
- 몽중식
- multiheadattention
- icml2024
- flowmatching
- vscode
- generativemodeling
- Theme
- loss-weighting
- diffusion
- deeplearning4science
- LLM
일 | 월 | 화 | 수 | 목 | 금 | 토 |
---|---|---|---|---|---|---|
1 | 2 | 3 | 4 | 5 | 6 | |
7 | 8 | 9 | 10 | 11 | 12 | 13 |
14 | 15 | 16 | 17 | 18 | 19 | 20 |
21 | 22 | 23 | 24 | 25 | 26 | 27 |
28 | 29 | 30 |